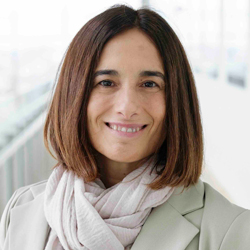
Carla Perrotta
Associate Professor, School of Public Health Physiotherapy and Sports Sciences, UCD
Associate Dean for Teaching and Learning, SPHSS, UCD
Director of Evidence Synthesis and Health Economics programmes, SPHSS, UCD
Director of Health Data Analytics, SPHSS, UCD
Evidence synthesis is essential in healthcare decision-making, and while AI can enhance efficiency, human expertise remains crucial for reliable decisions.
Evidence synthesis refers to the systematic process of gathering, evaluating and integrating data from scientific studies to answer healthcare-related questions. It is a fundamental part of current medical practice where decisions must be based on scientifically sound, transparent and reliable evidence.
Why is evidence synthesis important in healthcare?
From determining the effectiveness of yearly COVID-19 vaccinations to assessing exercise prescriptions in primary care to evaluating whether governments should fund a newly approved drug for cystic fibrosis, evidence synthesis is critical. It informs decision-making by providing recommendations based on an exhaustive analysis of the scientific evidence.
Yet, the rapid and continuous production of scientific research presents challenges. Today, studies are published globally at an unprecedented pace, making it difficult for methodologists and decision makers to keep up.
Evidence synthesis is not only time-consuming but also resource-intensive. For trained professionals to complete a systematic review takes 18 to 24 months on average. Translating this evidence into actionable decisions for healthcare policies and practices can take even longer, making the evidence-to-action process slower than the demands of modern healthcare.
How AI is changing evidence synthesis
Artificial Intelligence (AI), particularly through Natural Language Processing (NLP), is increasingly being explored as a tool to improve the evidence synthesis process. At the 2024 Global Evidence Summit in Prague, there was widespread interest in NLP’s potential. Presentations and discussions centred on the promise of Al for assisting with tasks like searching for and screening relevant studies, and extracting key data for analysis.
Evidence synthesis is not only time-consuming but also resource-intensive.
Al’s potential to automate repetitive tasks can significantly reduce time and effort expenditure during the evidence synthesis process. Properly trained algorithms are efficiently handling large volumes of research data and offering a way to manage the flood of information that characterises health research. But NLP has limitations. While Al performs well with binary tasks, it struggles with more complex tasks where context is crucial.
AI and human collaboration in evidence synthesis
Research teams, startups and other companies are investing heavily in integrating Al into the evidence synthesis process, suggesting that we will see more practical applications in time. Increased use of Al has the potential to improve the efficiency of evidence synthesis by managing vast amounts of data fast.
Still, human expertise remains essential. Only skilled professionals can design search strategies, anticipate potential bias, and appraise, integrate and interpret the evidence safely and transparently.